Abstract
Industrial control systems (ICS) are used to manage essential infrastructure such as power grids, water treatment plants, and transportation systems. These systems are critical in ensuring that our daily lives run smoothly and efficiently. However, with the increased use of ICS, cybersecurity has become a major concern. If attackers gain access to these systems, they can cause severe damage to equipment or infrastructure, harm the environment, and even risk people’s lives. It’s crucial to prioritize the security of ICS to prevent these potentially devastating consequences.
One of the challenges of securing ICS is that many ICS are built on legacy systems. These old systems were not designed with cybersecurity in mind, making them vulnerable to modern cyber threats. They often lack the necessary security features and protocols required to protect against such threats. As a result, it can be challenging to retrofit these systems with adequate security measures without disrupting their operations.
One way to protect ICSs against cyberattacks is by using advanced intrusion detection techniques that rely on machine learning algorithms. These algorithms continuously monitor the system’s networks, hosts, and physical processes to identify abnormal patterns that could indicate a cyberattack. By detecting such anomalies early, the algorithms can prevent significant damage from occurring. In this thesis, we explore how well different types of machine learning algorithms can be used to create effective intrusion detection systems. Additionally, we investigate to what extent the algorithms can distinguish different attack types.
However, testing intrusion detection methods on actual operating ICS can be dangerous and difficult. Therefore, researchers need controlled testbeds to test their methods. To this end, we introduce a framework for creating virtual testbeds that simulate real-world operational ICSs. This framework enables researchers to simulate various attack scenarios and study the effectiveness of different intrusion detection methods under different customized conditions. Moreover, we propose a set of simulated cyberattacks that can occur on ICSs. We use these attacks to validate and test different intrusion detection algorithms, as well as compare their performance.
List of publications in this thesis
Paper A: Digital Twin-based Intrusion Detection for Industrial Control Systems, Seba Anna Varghese, Alireza Dehlaghi Ghadim, Ali Balador, Zahra Alimadadi and Panos Papadimitratos. International Conference on Pervasive Computing and Communications (PerCom). Pisa, Italy, March 2022. (Published)
Paper B: ICSSIM – A Framework for Building Industrial Control Systems Security Testbeds, Alireza Dehlaghi-Ghadim, Ali Balador, Mahshid Helali Moghadam, Hans Hansson, Mauro Conti. Computers in Industry Jour nal, 2023. (Published)
Paper C: Anomaly Detection Dataset for Industrial Control Systems, Alireza Dehlaghi-Ghadim, Mahshid Helali Moghadam, Ali Balador, and Hans Hansson. (Submitted for publication)
Paper D: Time-series Anomaly Detection and Classification with Long Short Term Memory Network on Industrial Manufacturing Systems, Tijana Markovic, Alireza Dehlaghi-Ghadim, Miguel Leon, Ali Balador, Sasikumar Punnekkat. (Submitted for publication)
Advisors
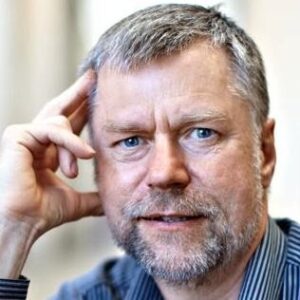
Main advisor
Hans Hansson
Professor
Mälardalen University
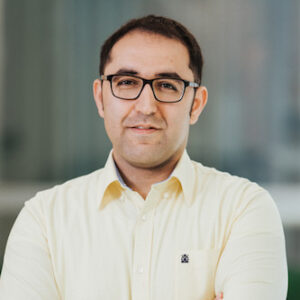
Co-advisor
Ali Balador
Assistant Professor
Mälardalen University
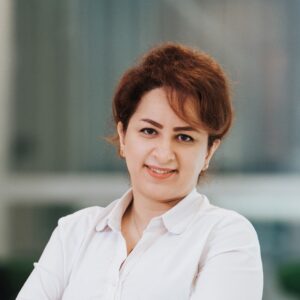
Co-advisor
Mahshid Helali Moghadam
PhD.
Data Scientist at Scania R&D
Examining Committee
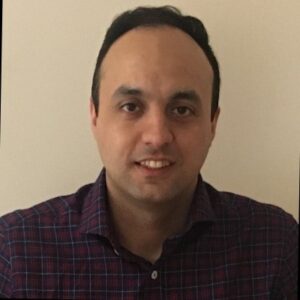
Mohammad Shojafar
Associate Professor
University of Surrey
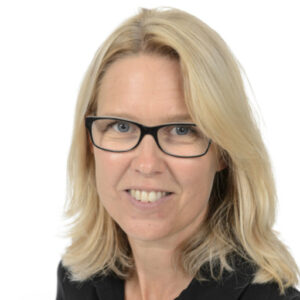
Maria Kihl
Professor
Lund University
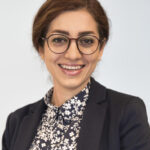
Tooska Dargahi
Assistant Professor
Manchester Metropolitan
University
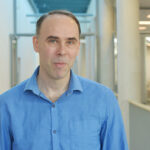
Johan Åkerberg
Assistant Professor
Mälardalen University